TC&C’s CEO, Csaba Juhász PhD and two colleagues from the University of Pannonia, Zsolt Vassy and István Vassányi conducted a noteworthy study which investigated the potential of using artificial intelligence methods (AI) on data generated from computer devices to diagnose illnesses. The findings are encouraging, particularly in regard to respiratory and mental diseases. Nevertheless, additional research is essential to arrive at definite conclusions, and it should involve a larger sample size.
You can read the full original article in Hungarian in this proceedings on pp 28-33.
AI-based behavioral analytics is proving useful in more and more areas. For example, TC&C’s CARIN QAi solution provides several tools to analyze customer sentiment and attitudes based on interactions in call centers, improving the customer experience and solving potential problems before they even arise. However, AI-based analytics does more than just help avoid customer experience flaws. It is also increasingly being used in the healthcare sector, where it helps prevent important problems by providing an early diagnosis. It is particularly useful in the area of disease diagnostics and risk prediction. AI can be used to assess existing data, aiding physicians in their decision-making. Deep learning systems are already employed in modern healthcare institutions in radiology due to their effective image recognition abilities.
What does your webcam say about you?
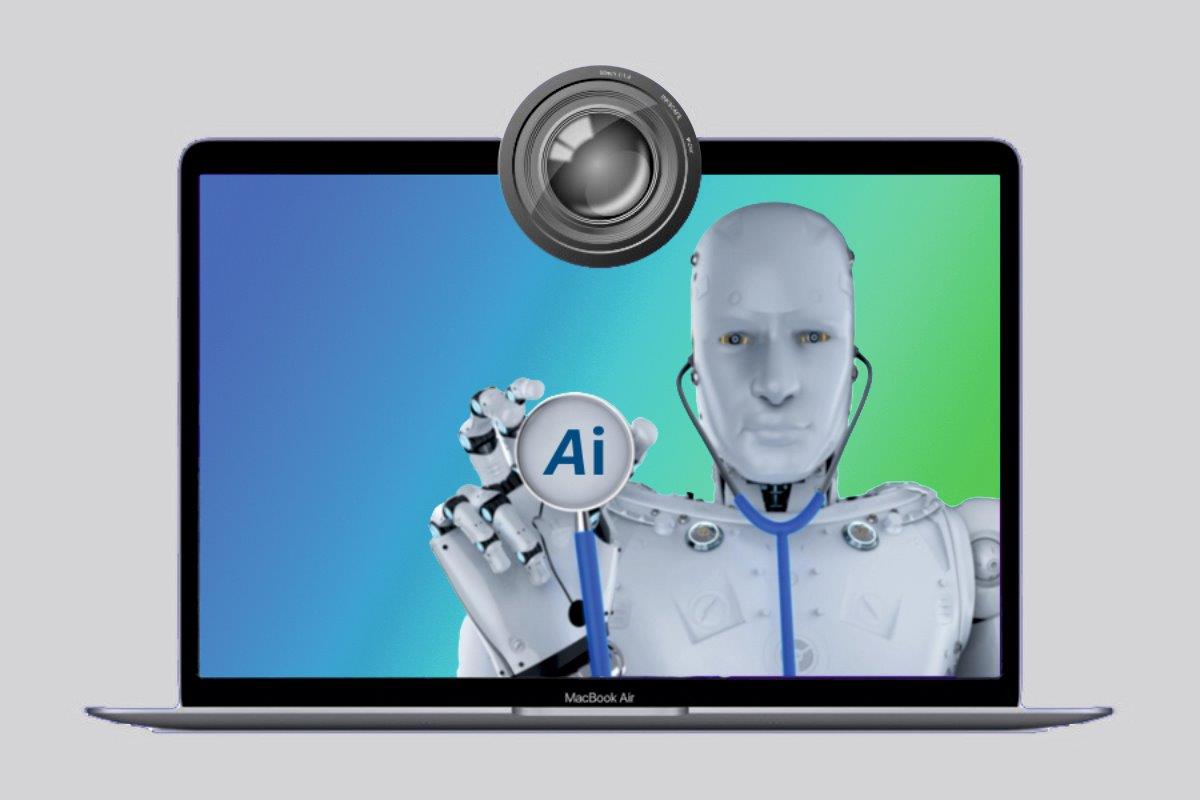
Other promising fields for exploration include the vast amount of data being generated and collected daily from home and office computers. In recent times, due to the pandemic, the amount of this data has multiplied as communication among people is recorded in audio and video form. How a person interacts with devices and the environment can be used to assess health. AI is capable of monitoring and analyzing these pieces of data and make predictions that can be of great importance for prevention.
While much of this data isn't recorded or shared, most enterprise quality assurance systems do have the capabilities to record it and many companies have started to use this option. Recording this data in corporate and educational settings is mostly possible, although the power requirements of the processing operation and data protection regulations can limit this. In the study, researchers explored the diagnostic potential of the data that is produced in everyday life in households and corporations.
Diagnosing the flu or COVID
Voice-based diagnostics used in the referenced studies harness the power of AI to monitor and identify any abnormalities in speech signals. It does this by analyzing the time, frequency, noise, disturbance and spectral structure of the speech recordings. Based on the evaluation of these features, the system can classify the recordings according to health conditions or other pathological categories.
Using this method, it is possible to detect the presence of an upper respiratory disease and even distinguish between different types of illness. For example, COVID-19 changes the voice and cough in a distinct way, altering speech features like acoustic waveform amplitude, speech frequency and cepstral peak. Additionally, formant mid-frequencies may be affected due to altered laryngeal movement and articulation.
Identifying brain disorders
Voice and speech-based diagnostics, as well as instrumentation-based diagnostics, can be used to identify mental illnesses or brain disorders. For example, depression and post-traumatic stress disorder can be diagnosed by machine recognition of features identified from the voice and matching up to hundreds of speech-sound features, finding 30-50 of them to diagnose the disease with high accuracy. Eye movement features can also be extracted from videos of faces to help diagnose depression.
In the case of Alzheimer's disease, AI and linguistic analysis combined can be used to detect the four clear factors - acoustic impairment, semantic impairment, syntactic impairment and information loss- to provide an early diagnosis.
Impressive results in a blink of an eye
Video analysis of facial features showed that there was no significant difference in blink rate between depressed and healthy subjects. However, compared to healthy individuals, those with depression had a significantly smaller average distance between their eyelids (i.e. degree of eye opening) and a significantly longer average duration of blinks - likely due to fatigue or the avoidance of eye contact. Utilizing these features, Machine Learning models have been demonstrated to detect depression with an accuracy of 70-75%. Post-traumatic stress syndromes were screened for with a success rate of 89.1% based on speech patterns. Additionally, using biomarkers from speech, word use, and tool use, Alzheimer's Disease can be identified, on average, 7 years earlier with an accuracy of over 70%.
Bright future with a few issues to address
Automatic evaluation using AI can be useful for remote, non-contact diagnostics. One advantage of these methods is that they can be used without disrupting daily activities. They can also be used for low-cost and non-invasive mass screening and diagnosis, even utilizing common devices in the course of daily life.
However, important issues need to be addressed and further research is necessary, to make sure that these technologies can be safely and effectively applied. For example, the present scientific research has only used a small number of categorized media (samples of 100-300) to teach and control learning systems, which is an insufficient amount for AI technologies. Tens of thousands of samples would be needed to validate these methods.
Additionally, privacy is an important factor: these technologies may raise serious privacy concerns, such as the possibility of identifying individuals from anonymous speech samples or accessing and misusing sensitive medical information.